Running multimodal LLMs on-device sounds like a great idea.
It's good for privacy, good for latency, doesn't need internet and it's free compute. There's a problem though: many devices barely have enough resources to fit a small LLM, and not a smart one. A tiny LLM can still do many things: talk, recognize text, reason, segment images - just not all at the same time.
That is, not unless we help it out with hot-swappable adapters.
Three tasks vs. one model
Consider a chore such as sorting your trash.
A prime example of a "why can't we use AI for this?" candidate for automation. You have to do it all the time, the rules are confusing and vary by community, and the whole process just begs to be replaced by a phone app.
Let's outline the design of such an app. We could use instance segmentation to identify and highlight different kinds of trash. In order to find out where each item goes, we need to refer to local waste disposal rules. Finally, we could highlight important quirks like garbage day schedule or district-specific trash bags. We can do it all using a single PaliGemma1 base model by plugging in a task-specific adapter2 for every step.
Could we do it using a YOLO and a couple regular expressions? Probably, if we wanted to disturb our old wounds inflected by poor domain generalization, imbalanced data and open class classification. At least PaliGemma is relatively huge, so we can hope it has enough world knowledge to make these issues go away.
With this overview in mind, let's move on to see how the sausage is made.
Segmentation by day, text by night
Check out the full demo on GitHub.
Fundamentally PaliGemma is a model designed to generate text token by token.
In order to teach it to output segmentation, we need find a way to represent bounding boxes and masks as sequences of tokens. After that, we need to train the model using obscene amounts of data, and then it will hopefully learn how to segment an image.
Luckily, Google's already done the hard part. For PaliGemma, each segmentation mask is represented by a sequence of 20 special tokens. First four of them look like <loc0000>
and contain bounding box coordinates. Remaining 16 tokens look like <seg000>
and encode the mask as a vector of numbers between 0 and 127. In order to move between this 16-token representation and the original mask we use a special pretrained autoencoder3. Class labels are included at the end as plain text.
JSON, of course, is already text, so it hardly needs special treatment. However, following a notebook for Idefics 24 I decided to translate JSON labels into an XML-like format. I assume this helps the model to keep track of nested structures by replacing endless curly braces with unique tags.
The rest of the project is more in line with regular LLM stuff, and is best explained with some hands on examples.
Garbage gymnastics
The practical part begins with gathering the data.
I decided to go with a small instance segmentation dataset called TACO5. It's reasonably clean and looks kind of like what we need.
The first adapter is going to do instance segmentation. Our dataset comes with annotations for 60 different classes, but we are going merge them into a total of 9. We also need to turn masks into text as discussed in the previous section.
The job of the second adapter is to cross-reference segmented items with local waste disposal rules and produce instructions in JSON format. We need to generate some synthetic data for this. Let's pluck label names out of our dataset, combine them with rules6 and use GPT-4o to generate target JSONs. During training we are going to input segmented images, labels and rules, and ask the model to predict the target part.
Now let's see what the model in question thinks of our data.
The easiest part of the project
Implementing all the parts we talked about earlier would surely require some elbow grease.
However, we are going to skip that completely by using the TensorSense Training Toolkit. This is a library I made that comes with tools for the gnarliest parts of our project.
In order to start training, we need to convert both of our datasets into a HuggingFace Datasets7 format. We will also create a custom collator8. This is a utility that turns samples into actual LLM input tensors. Luckily, the rest of our workflow is not data-dependent, meaning we can use generic presets from the library. Let's finish the setup by plugging our parts into the factory to get a Trainer
for the model.
Cooking each of our adapters should take around 20 minutes. Once they are done, we can load both of them together with the base model to get our memory efficient AI system rolling. Now we can call those adapters one after another and get the results.
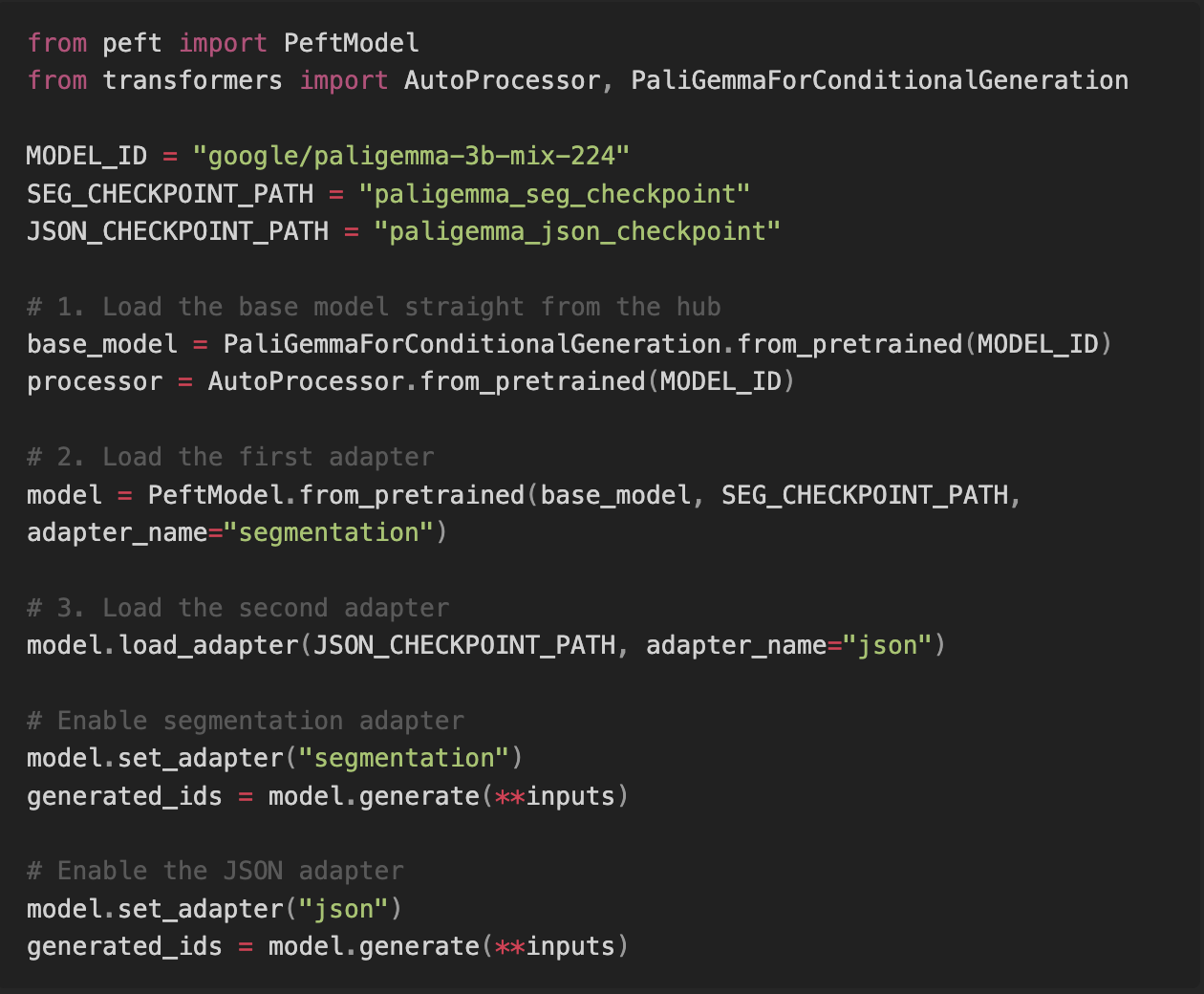
Enjoy your trash sorting!
P. S. If you would like to see the gory details of this project, take a look at the full demo on GitHub.
Hi! Andrey here.
Thanks for making it till the end! If you enjoy reading Make AI, please consider subscribing to the newsletter. It helps a lot!
Either way, thanks for reading, and I’ll see you in the next one!
PaliGemma – Google's Cutting-Edge Open Vision Language Model. This is a 3B multimodal LLM that has been pretrained not only on question answering, but also on OCR, object detection and instance segmentation. This is the reason we're picking it over other similar models.
Adapters on HuggingFace PEFT. This is a method of fine-tuning by injecting a small set of trainable parameters into a frozen base transformer. Since training an adapter does not affect original weights, we can switch between multiple adapters for the same model during inference without having to reload the whole thing.
Ning et al.. We use a pretrained version of this model to encode segmentation masks into 16-dimensional quantized vectors (meaning they contain integer values from 0 to 127) and back.
The notebook in question. It's also been adapted to different other models with varying degree of success.
I gathered a few examples of such rules by manually googling trash sorting rules in different cities across the globe.
You can find the code for the collator in this notebook in the full demo repository.